Shanghai University
Article Information
- Hai-jun PENG, Qiang GAO, Hong-wu ZHANG, Zhi-gang WU, Wan-xie ZHONG. 2014.
- Parametric variational solution of linear-quadratic optimal control problems with control inequality constraints
- Appl. Math. Mech. -Engl. Ed., 35(9): 1079-1098
- http://dx.doi.org/10.1007/s10483-014-1858-6
Article History
- Received 2013-9-6;
- in final form 2014-2-19
2. State Key Laboratory of Structural Analysis for Industrial Equipment, School of Aeronautics and Astronautics, Dalian University of Technology, Dalian 116024, Liaoning Province, P. R. China
The linear-quadratic (LQ) optimal control problem has always been viewed as the fundamental problem of the optimal control theory. It is usually used as an important tool for practical aerospace control engineering. Its applications include planning spacecraft rendezvous utilizing a finite low thrust,satellite formation flying,station keeping and/or reconfiguration technology,and reentry vehicle trajectory guidance. Indeed,the maximum thrust and the momentum provided by the engine are both finite. Therefore,the LQ optimal control problem with control inequality constraints is commonly encountered and difficult to be solved.
From the feedback control law viewpoint,Kojima and Morari [1] introduced singular value decomposition for the finite time horizon linear systems,and obtained the sequence of LQ sub-optimal control laws,which was converged to an exact solution,based on quadratic programming. Bemporad et al. [2] proposed an explicit solution for the model predictive control based on a linear programming method. To decrease the online computation work of the model predictive control,Bemporad et al.[3] solved the explicit solution of the feedback gain for the LQ optimal control with state and control constraints. Goebel and Subbotin [4] studied a continuous-time infinite-horizon linear quadratic regulator with input constraints.
From the trajectory optimization viewpoint,the numerical methods used to solve the optimal control problem can be divided into two major classes: the indirect methods and the direct methods [5, 6] . The indirect methods can transform the optimal control problem into a Hamiltonian boundary value problem (HBVP) by the calculus of variations or the maximum principle. The obtained HBVP can then be solved by the numerical methods such as the shooting method [7] and the multiple-shooting method [8] . The primary advantages of the indirect methods are the high accuracy in the solution and the assurance that the solution satisfies the first-order optimality conditions. However,the indirect methods have several disadvantages, including the small radii of convergence and the need to analytically derive the HBVP and an initial guess for the costate [9] . With the direct methods,the optimal control problem can be discretized and converted into parameter optimization problems,which can be solved by a nonlinear programming method [10, 11] . With different discretization schemes for the control and state variables,various direct methods are derived,e.g.,the local collocation methods [12, 13] , the global collocation methods [14, 15] ,and the time finite element methods [16, 17] . The direct methods are more advantageous than the indirect methods because they have larger radii of convergence and require no initial guess for the costate. However,for most direct methods,the solutions do not satisfy the first-order optimality conditions,and the costate variables cannot be estimated.
The present work involves conducting a numerical analysis of an LQ optimal control problem with control inequality constraints by using an adjustable or a variable parameter multiplier. The concept of a variable parameter multiplier was first introduced by Zhong and Zhang [18] to obtain the numerical solutions for elastoplastic structures and contact problems. In this paper, the variable parameter multiplier theory is generalized to develop a new numerical approach for analyzing the LQ optimal control problems with control inequality constraints. In the development of the approach,two fundamental issues have been addressed. First,a variable parameter is introduced to replace the inequality constraints by equality constraints. Under the equality constraints of the LQ optimal control problem,the parametric variational principle can be used to derive the Hamiltonian canonical equations coupled with the linear complementarity equations. Second,because the numerical solutions of the LQ optimal control problem can only be obtained at discrete-time points,the continuous time domain can be divided into a series of intervals. The linear complementarity solver is used to solve the variable parameters for all discrete-time points. In the proposed method,the control variable is expressed explicitly by the parametric variables,whereas in the indirect method,the control variable is determined implicitly by minimizing the Hamiltonian function. Nonetheless,the linear complementarity problem obtained in this paper is different from the programming problem obtained by direct methods. The solution of the proposed method satisfies the Hamiltonian canonical equations and provides the costate information.
The pseudospectral method for solving optimalcontrol problems has been studied and used widely from theory to practical applications [9, 14, 15, 19, 20, 21, 22, 23, 24] recently,and more and more atten-tion has been paid to it. A pseudospectral method,which belongs to the global collocation methods,is typically used in a single mesh interval,and the convergence is achieved by increasing the degree of the polynomial. Some pseudospectral methods can also be used to establish the costate mapping theorem,and the costate variables can be estimated by the Lagrange multipliers[9, 14, 15] . For problems where the solutions are infinitely smooth and well behaved, a pseudospectral method has a simple structure and converges at an exponential rate [19, 20] . However,the convergence rate of a pseudospectral method for a problem whose formulation or solution is nonsmooth may be extremely slow,resulting in a poor approximation even when a high-degree polynomial is used [19, 20, 21, 22] . The pseudospectral method exhibits the well-known Gibbs phenomenon,resulting from the approximation of a nonsmooth function by a finite number of smooth functions [21, 23] . In addition,the limitation of a global pseudospectral method is that the use of high-degree global polynomials results in a nonlinear programming problem (NLP) for which the density grows quickly as a function of the number of the NLP variables. Thus,a global pseudospectral method becomes computationally intractable for the problems when an excessively large-degree polynomial is required [19, 20] .
For an LQ optimal control problem with control inequality constraints,the solutions of control variables are nonsmooth. Unlike the global pseudospectral method,variable parameter multipliers are used in many mesh intervals,and the solutions of the LQ optimal control problem are determined by these local variable parameter multipliers. Therefore,the well-known Gibbs phenomenon will not occur,and the convergence of the proposed method,which will not be influenced by the nonsmooth character,is achieved by increasing the number of the mesh intervals. Meanwhile,the costate variables can be evaluated by the variable parameter multipliers,and then the first-order optimality conditions can be checked. In addition,with the increase in the mesh intervals,the linear complementarity problem formulated by the proposed method is sparse. Thus,the computational efficiency of a sparse problem is better than that of a density problem with the same number of mesh intervals. The new low-order variable adaptive techniques [19] and the new mesh refinement techniques [22] are developed to increase the ability of pseudospectral methods. 2 LQ optimal control problems with control inequality constraints
The LQ optimal control problems with control inequality constraints in a finite continuoustime domain are considered in this paper. The linear differential equation for a controlled system can be expressed in the form of a state space as follows: ˙
where



For an LQ optimal control problem,the boundary condition at the terminal time is
For an LQ optimal control problem with hard terminal constraints,the terminal boundary condition is
where xf is the given terminal state. In the following sections,a parametric variational principle and the corresponding numerical algorithm are proposed to solve the LQ optimal control problem with control inequality constraints. 3 Parametric variational principle for LQ optimal control problems with control inequality constraintsIn this section,based on the parametric variational principle [18, 25, 26] ,theLQoptimalcontrol problem with control inequality constraints is transformed into the Hamiltonian canonical equations coupled with the linear complementarity equation. The parametric variational principle can be used to overcome the limitations of the classical variational method [18, 25, 26] and address the inequality constraints.
By introducing the variables and
,Eq. (2) can be transformed into the following constraints:








Because the computation of the parametric variables and
is essential to solve the
LQ optimal control problem with control inequality constraints,the next section focuses on
numerically determining the parametric variables by dividing the continuous time into a series
of discrete intervals with the linear complementarity solver. The state variablex,thecostate
variable λ,and the control variableucan be obtained through the known parametric variables.
Note that the above procedure is different from the Hamiltonian boundary value problem derived by the indirect method. In the indirect method,the control variable is determined implicitly by minimizing the Hamiltonian function,while the control variable is expressed explicitly by the parametric variables in this paper. 4 Numerical method to solve coupled Hamiltonian canonical equations and linear complementarity problem
In the previous section,the coupled Hamiltonian canonical equations and the linear complementarity equations are established. These coupled equations are difficult to be analytically solved in a continuous time domain. Therefore,in this paper,the continuous time is divided into a series of time intervals,and the state variables,the costate variables,and the control variables are solved on these discrete time points.
The Hamiltonian canonical equations (14) and (15) can be rewritten as
where The solution of the linear ordinary differential equation (20) can be expressed by where u0 is the initial value of the ordinary differential equation,and Φk(·,·) denotes the transfer matrix.For the numerical simulation,the continuous-time domain is divided into a series of intervals of equal length,i.e.,
whereηis the length of a time interval,and Nis the number of the intervals. In this paper, within a time interval [tk−1,tk](k=1,2,···,N),the parametric variables







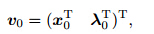





For the LQ optimal control problem with hard terminal constraints,the linear complementarity problem is the same as that of Eqs. (43)-(53) except forM1andqwhich are now given by
Thus,the coupled Hamiltonian canonical equations and the linear complementarity equations can be transformed into a linear complementarity problem of Eqs. (43)-(45). The linear complementarity problem can be solved by the pivotal method [27] ,the interior point method [28] , etc. In this paper,the Lemke algorithm,which belongs to the pivotal method category,is used to solve the linear complementarity problem.Note that the linear complementarity problem of Eqs. (43)-(45) can be obtained by assuming that the continuous-time linear complementarity problem of Eqs. (16)-(19) is satisfied at the right point of each time interval. This is not the only choice. The similar linear complementarity problem can also be derived if the continuous-time linear complementarity problem of Eqs. (16)- (19) is satisfied at the left or at the middle point ofevery time interval. Moreover,the parametric variables in every time interval can be linearly approximated to derive the discrete-time linear complementarity problem.
For the LQ optimal problems with control inequality constraints,by solving the linear
complementarity problem with the famous Lemke algorithm,the parametric variables and
in all time intervals can be obtained. Then,the state,costate,and control variables can be
computed directly. For the LQ optimal control problems,the state and the costate variables
are given by Eqs. (28) and (34),respectively,and the control input is given by Eq. (11). For the
LQ optimal control problems with hard terminal constraints,the state and costate variables
can be computed by Eqs. (28) and (35),respectively,and the control input is also determined
by Eq. (11).
It is important to note that the linear complementarity problem obtained in this paper is different from the quadratic programming (QP) problem obtained by the local direct collocation method for the LQ optimal control problem. The solution of the linear complementarity problem satisfies the Hamiltonian canonical equations,and it gives the costate information that can be used for the error analysis and solution verification. However,the solution of the local direct collocation method does not satisfy the first-order necessary condition of the optimal control, and it is uncertain whether the direct solution of the QP problem is truly optimal for the original continuous LQ optimal control problem [29] . 5 Computation of transfer matrix and related integration
In Section 4,to solve the LQ optimal control problem with control inequality constraints, one of the main problems is to calculate the transfer matrix and its related integration,i.e., Eqs. (29) and (30). The calculation of the transfer matrix can be divided into two categories: the time-invariant system (i.e.,the coefficient matrices of the system equation,Eq. (1),and the performance index,Eq. (3),are independent of time) and the time-varying system (i.e.,the coefficient matrices of the system equation and the performance index are related to time).
For the time-invariant systems,the coefficient matrices A(t) and B(t) of the system equation,Eq. (1),and the weighted matrices Q(t)and R(t) of the performance index,Eq. (3),are constant matrices. Therefore,the matrices Ak,Bk,Qk,and Rk(k=1,2,···,N)areallthe same. Moreover,it is easy to prove that,for time-invariant systems,the corresponding transfer matrix only depends on the matrix and the length of the interval. Thus,the transfer matrix and its related integration,which are given by Eqs. (1) and (3),can be simplified as
where exp (·) denotes the matrix exponential. In general,the matrix exponential in Eq. (56) can be computed by the expm function in the MATLAB software. However,to achieve better precision,the precision integration method is used in this paper to compute the matrix exponential.For the time-varying systems,the coefficient matricesA(t)andB(t)ofEq.(1)andthe weighted matrices Q(t)and R(t) of Eq. (3) depend on time,and the precision integration method cannot be used directly to compute the transfer matrix and its integration. In this paper,the Magnus series method [30, 31] ,a structure-preserving numerical algorithm,is used to calculate the transfer matrix for the time-varying systems.
The transfer matrix of the time-varying systems can be expressed by the matrix exponential of the Magnus series,i.e.,
where the Magnus series Ω(t,0) is defined as in which
Iserles and Nørsett [30] studied the general form of the Magnus series. With a two-stage Gauss quadrature formula to Eq. (58),they gave an approximate formula for the Magnus series. According to their results,in a time interval [tk−1,tk],the transfer matrix can be given as the matrix exponential of an approximation of the Magnus series[30, 31] ,i.e.,
where The numerical method given above is a fourth-order formula. By applying a higher-order quadrature formula to Eq. (58),other approximations with higher-order terms for the Magnus series can be obtained. The advantage of the Magnus series method is the preservation of the qualitative properties of the real solutions [31] .According to the property of the transfer matrix,the transfer matrix and its related integration can be expressed as
Therefore,the transfer matrix Φkk,0 of the time-varying system can be calculated by Eqs. (63) and (59),and the matrix Ψk,ican be calculated from Eqs. (59) and (64) by the Gauss integration formula.Once the transfer matrix is obtained,the LQ optimal control problem with control inequality constraints can be solved by the numerical method given in Section 4. 6 Implementation and results 6.1 Multilevel implementation of parametric variational algorithm
In the previous sections,the parametric variable method and the corresponding numerical algorithm are given for the LQ optimal control problem with control inequality constraints. However,detailed implementations for practical applications are worth considering. In this paper,a multilevel iteration approach is adopted to improve the efficiency of the numerical method.
The main idea of the multilevel iteration is as follows. The efficiency of solving the linear complementarity problem highly depends on the initial iteration values. Therefore,choosing better initial values is essential. In this paper,the LQ optimal control problem is first solved on a sparse time grid,and then the initial values of the parametric variable multipliers,which are used to solve the linear complementarity problem on dense time grids,are approximated by the parametric variable multipliers on the sparse grid. The details of the implementation are given as follows:
(i) Assume that the fixed time length of the LQ optimal control problem is tf,andthe solutions on the grids with 2Mequal subintervals are required.
(ii) Divide the whole time interval into 2M0
(M0 (iv) Solve the LQ optimal control problem with control inequality constraints by the parametric variable algorithm proposed in this paper,and obtain the parametric variable multipliers
and the state and costate variables.
(v) If the number of grids satisfies the requirement of Step (i),then stop the computation;
otherwise,double the time grids,i.e.,divide the whole time interval into 2m+1 subintervals with
equal length,and letm:= m+1.
(vi) Map the parametric variable multipliers of the sparse grids (themlevel) onto the dense
grids (m+1 level) with the Lagrange interpolation.
(vii) Solve the linear complementarity problem on the dense grids (them+1 level) by the
parametric variable multipliers on the dense grids as the initial values of the Lemke algorithm.
(viii) Go to Step (v).
For the above adaptive algorithm,when the grids are sparse,the scale of the linear complementarity problem is small. Therefore,it is efficient to solve the linear complementarity
problem. When the time grids are refined,the initial values used in the Lemke algorithm are
approximated by the results of the sparse grids,which are closer to the analytical solution.
Therefore,on the fine grids,the convergencespeed of the Lemke algorithm is much faster.
6.2 Numerical examples
First,two numerical examples with time-invariant and time-varying coefficient matrices are
used to test the precision and the efficiency ofthe proposed algorithm. Then,the numerical
algorithm is used to solve an optimal control problem of rendezvousing spacecrafts with a finite
low thrust.
To test the precision of the proposed algorithm,the relative errors of the state and the
control variables are defined as follows:
For the first two examples,the proposed method is compared with the direct collocation
method and the pseudospectral method. In the detailed implementation of the direct collocation method,an explicit Euler scheme and an implicit trapezoidal scheme are used to discretize the state and control variables. The LQ optimal control problem with control inequality constraints is transformed into a standard quadratic programming problem which is solved by
the subroutine quadprog of MATLAB. A Radau pseudospectral method
[32]
is also used for the
numerical comparisons in this paper. The pseudospectral method is used widely in nonlinear
trajectory optimization,and needs the computation of the derivatives of the objective function
gradient and the Jacobian matrix for increasing the efficiency of the nonlinear program. In
this paper,“pseudospectral method 1” means that the finite differencing method is used to
compute the gradient and the Jacobian matrix,and “pseudospectral method 2” denotes that
the analytic derivative is used for the gradient and the Jacobian matrix.
Example 1 A one-dimensional time-invariantsystem is considered. The differential equation and the initial condition of the controlled system are
Figures 1 and 2 give the numerical results for the state,costate,and control variables
when 32 intervals are used,which show that theyare very close to the reference solutions. In
particular,Fig. 2 shows that the control constraint given in Eq. (68) is satisfied. Furthermore,
for different intervals,i.e.,23,24,···,210,the proposed method is compared with the Euler
collocation method and the trapezoidal collocation method. The precision and CPU time for
the proposed method,the Euler collocation method,the trapezoidal collocation method,and
the pseudospectral methods are given in Figs. 3 and 4. It can be seen that the pseudospectral
method has the fastest convergence rates among all the numerical methods. However,if the
same CPU time is used,the proposed method is more accurate than the Euler collocation
method,the trapezoidal collocation method,and the pseudospectral method. To achieve the
same precision,the proposed method needs less CPU time than the other methods.
Example 2 In this example,a one-dimensional time-varying system is considered
[33, 34]
.
The time-varying differential equation and the initial/terminal conditions of the controlled
system are
The numerical results computed by the proposed method using 64 equal intervals are given
in Figs. 5 and 6,which show that the numerical results of the state,costate,and control variables agree with the exact solutions. Figures 7 and 8 give the efficiency and the precision
comparisons among the proposed method,the Euler collocation method,the trapezoidal collocation method,and the pseudospectral method. The conclusions from these two figures are
the same as those in Example 1,i.e.,the pseudospectral method has the fastest convergence rates among all the numerical methods. However,if the specific precision or CPU time is given,
the proposed method and the pseudospectral methods are more advantageous than the other
methods. Especially,the proposed method ismore accurate than the pseudospectral method
for control variables.
Example 3 In this example,an optimal control problem for rendezvousing spacecrafts with
a finite low thrust is considered. Because theorbits of the spacecrafts may be circular or
elliptical,the relative dynamical linearization equation describing the rendezvous can lead to
the Clohessy-Wiltshire (C-W) equation for time-invariant systems or the Lawden equation for
time-varying systems. Therefore,in the following paragraphs,the optimal control problem
is discussed based on these two different kinds of relative dynamical models. The reference
coordinate (X,Y,Z) with its origin at the center of the Earth is the inertial coordinate system,
and the relative coordinate frame (x,y,z) with its origin on the lead spacecraft is the main
coordinate frame for the relative dynamical equation. The x-axis is along the radius from
the Earth to the leader spacecraft,they-axis is in the direction of motion,and the z-axis is
perpendicular to the orbit plane to complete a right-handed coordinate system.
For the circular reference orbit,the linearization equation of the relative dynamics is the
well-known time-invariant C-W equation
[35]
,which can be expressed in the form of a state space as follows:
For the elliptical reference orbit,the leader spacecraft follows an elliptical reference trajectory
with the Earth’s center at one of its foci. The linearization equation of the relative dynamics is
the well-known time-varying Lawden equation
[36]
,whichcanalsobeexpressedintheformofa
state space as follows:
For both the time-invariant C-W equation and the time-varying Lawden equation,the optimal control problem of rendezvousing spacecrafts is the minimization of the cost expenditure,
i.e.,the performance index is selected as
In this example,the initial state is given as
(5 000 m,−3 000 m,4 000 m,−30 m/s,−10 m/s,20 m/s)T Second,the velocity increments for differentrendezvous times are discussed for the timevarying Lawden equation. Three cases with different control constraints ulimit are considered:
Case 1,ulimit =0.07 m/s2,Case 2,ulimit =0.08 m/s2,and Case 3,ulimit =0.09 m/s2.The
eccentricityefor the Lawden model is 0.5,and the other parameters are the same as those
given before.
In Fig. 9,the velocity increments ΔV(m·s-1) for the three types of control constraints are
shown with different rendezvous time. The results show that the velocity increments are significantly different before 1 200 seconds,and converge after 1 300 seconds. This phenomenon
can be explained as follows. If the rendezvous time is long enough,the optimal controls cannot
reach the limitation of the three control constraint types. Therefore,the rendezvousing spacecraft problems corresponding to the three types of control constraints all become unconstrained
thrust rendezvous problems,and the control constraints have no influence on the velocity increments. Figure 9 also shows that for fixed rendezvous time,if the constraint of the thrust acceleration is stricter,the velocity increments are larger,and for thesame constraint on the
thrust acceleration,the smallestvelocity increments exist for different rendezvous time,i.e.,the
A,B,andCpoints given in Fig. 9.
Figures 10 and 11 give the detailed thrust accelerations,the relative positions,and the
relative velocities for the rendezvous time of 1 000 seconds and 1 500 seconds,respectively.
Table 2 gives the smallest velocity increments and the rendezvous time for three different
control constraints,which show that the smallest velocity increments correspond to different
rendezvous time and that the stricter constraints on the thrust acceleration lead to longer
rendezvous time corresponding tothe smallest velocity increment.
Based on the parametric variational principle,the LQ optimal control problem with control
inequality constraints is transformed into the Hamiltonian canonical equations coupled with
the linear complementarity equations. The linear complementarity problem is solved by the
linear complementarity solver in the discrete time domain. The algorithm proposed in this
paper is suitable not only for time-invariant systems but also for time-varying systems. To
improve the efficiency of solving the linear complementarity problem,a multilevel iteration
idea is proposed in this paper. The numerical examples indicate that the proposed method is
effective for the LQ optimal control problem withcontrol inequality constraints. The proposed method is successfully used to solve an optimalcontrol problem of rendezvousing spacecrafts
with a finite low thrust.
Fig. 1 State and costate trajectories
Fig. 2 Time history of optimal input
Fig. 3 State precision and efficiency comparison of different methods
Fig. 4 Control precision and efficiency comparison of different methods
Fig. 5 State and costate trajectories
Fig. 6 Time history of optimal input
Fig. 7 State precision and efficiency comparison of different methods
Fig. 8 Control precision and efficiency comparison of different methods denote the relative velocities in the x,y,and z-directions,and ux,uy,
and uz are the three components of the thrust acceleration and are taken as the control inputs.
are the limits of the thrust acceleration in the x-,y-,and zdirections,respectively. To measure the cost expenditure for rendezvousing spacecrafts,the
velocity increments are defined as
[37]
First,the velocity increment for different control constraints is considered for both the CW and Lawden equations. The rendezvous timeT is 1 000 s,the length of the semimajor
axis is 10 000 km,and the limits of the thrust acceleration in the x-,y-andz-directions are
assumed to be the same and are denoted with the symbolu
limit (in unit of m·s-2). The
velocity increment ΔV (in unit of m·s-1) is computed for five different control constraints: ulimit =0.06,0.07,0.08,0.09,infinite,in which “infinite” indicates that there is no constraint
used to the control input. To solve this problem,256 intervals with equal length are used,and
the results are given in Table 1. Table 1 shows that there is no difference between the velocity
increments ΔV for the time-invariant C-W model and the time-varying Lawden model when
the orbit is a circular orbit,i.e.,when the eccentricity is zero. From the theoretical analysis,
the C-W model is equivalent to the Lawden model when the eccentricity is zero. Hence,the
numerical results are consistent with the theoretical analysis,which proves the correctness of
the proposed method in certain contexts. Table 1 also shows that for a fixed rendezvous time,
the velocity increments increase when the limits on the thrust acceleration ulimit decrease,
indicating that the optimal solution obtained by the finite low thrust is the suboptimal solution
of the unconstrained optimal spacecraft rendezvous.
Fig. 9 Velocity increments versus rendezvous time for three different control constraints
Fig. 10 Numerical results of rendezvousing spacecrafts for rendezvous time of 1 000 s
Fig. 11 Numerical results of rendezvousing spacecrafts for rendezvous time of 1 500 s
[1] | Kojima, A. and Morari, M. LQ control for constrained continuous-time systems. Automatica, 40, 1143-1155 (2004) |
[2] | Bemporad, A., Borrelli, F., and Morari, M. Model predictive control based on linear programming- the explicit solution. IEEE Transactions on Automatic Control, 47, 1974-1985 (2002) |
[3] | Bemporad, A., Morari, M., Dua, V., and Pistikopoulos, E. N. The explicit linear quadratic regulator for constrained systems. Automatica, 38, 3-20 (2002) |
[4] | Goebel, R. and Subbotin, M. Continuous time linear quadratic regulator with control constraints via convex duality. IEEE Transactions on Automatic Control, 52, 886-892 (2007) |
[5] | Betts, J. T. Survey of numerical methods for trajectory optimization. Journal of Guidance, Control, and Dynamics, 21, 193-207 (1998) |
[6] | Betts, J. T. Practical Methods for Optimal Control Using Nonlinear Programming, Society for Industrial and Applied Mathematics, Philadelphia (2001) |
[7] | Hull, D. G. Initial lagrange multipliers for the shooting method. Journal of Guidance, Control, and Dynamics, 31, 1490-1492 (2008) |
[8] | Lenz, S. M., Bock, H. G., Schlöder, J. P., Kostina, E. A., Gienger, G., and Ziegler, G. Multiple shooting method for initial satellite orbit determination. Journal of Guidance, Control, and Dynamics, 33, 1334-1346 (2010) |
[9] | Benson, D. A., Huntington, G. T., Thorvaldsen, T. P., and Rao, A. V. Direct trajectory optimization and costate estimation via an orthogonal collocation method. Journal of Guidance, Control, and Dynamics, 29, 1435-1440 (2006) |
[10] | Hull, D. G. Conversion of optimal control problems into parameter optimization problems. Journal of Guidance, Control, and Dynamics, 20, 57-60 (1997) |
[11] | Biegler, L. T. Nonlinear Programming: Concepts, Algorithms, and Applications to Chemical Processes, Society for Industrial and Applied Mathematics, Philadelphia (2010) |
[12] | Margraves, C. R. and Paris, S. W. Direct trajectory optimization using nonlinear programming and collocation. Journal of Guidance, Control, and Dynamics, 10, 338-342 (1987) |
[13] | Tang, S. and Conway, B. A. Optimization of low-thrust interplanetary trajectories using collocation and nonlinear programming. Journal of Guidance, Control, and Dynamics, 18, 599-604 (1995) |
[14] | Fahroo, F. and Ross, I. M. Costate estimation by a Legendre pseudospectral method. Journal of Guidance, Control, and Dynamics, 24, 270-277 (2001) |
[15] | Gong, Q., Ross, I. M., and Fahroo, F. Costate computation by a Chebyshev pseudospectral method. Journal of Guidance, Control, and Dynamics, 33, 623-628 (2010) |
[16] | Warner, M. S. and Hodges, D. H. Solving optimal control problems using hp-version finite elements in time. Journal of Guidance, Control, and Dynamics, 23, 86-94 (2000) |
[17] | Estep, D. J., Hodges, D. H., and Warner, M. The solution of a launch vehicle trajectory problem by an adaptive finite-element method. Computer Methods in Applied Mechanics and Engineering, 190, 4677-4690 (2001) |
[18] | Zhong, W. X. and Zhang, R. L. Parametric variational principles and their quadratic programming solutions in plasticity. Computers and Structures, 30, 887-896 (1988) |
[19] | Darby, C. L., Hager, W. W., and Rao, A. V. Direct trajectory optimization using a variable low-order adaptive pseudospectral method. Journal of Spacecraft and Rockets, 48, 433-445 (2011) |
[20] | Darby, C. L., Hager, W. W., and Rao, A. V. An hp-adaptive pseudospectral method for solving optimal control problems. Optimal Control Applications and Methods, 32, 476-502 (2011) |
[21] | Ross, I. M. and Fahroo, F. Pseudospectral knotting methods for solving optimal control problems. Journal of Guidance, Control, and Dynamics, 27, 397-405 (2004) |
[22] | Gong, Q., Fahroo, F., and Ross, I. M. Spectral algorithm for pseudospectral methods in optimal control. Journal of Guidance, Control, and Dynamics, 31, 460-471 (2008) |
[23] | Guo, T., Jiang, F. H., and Li, J. F. Homotopic approach and pseudospectral method applied jointly to low thrust trajectory optimization. Acta Astronautica, 71, 38-50 (2012) |
[24] | Fahroo, F. and Ross, I. M. Advances in pseudospectral methods for optimal control. AIAA Guidance, Navigation and Control Conference, AIAA 2008-7309, Hawaii (2008) |
[25] | Ding, H. L., Yang, B. E., Lou, M., and Fang, H. F. New numerical method for two-dimensional partially wrinkled membranes. AIAA Journal, 41, 125-132 (2003) |
[26] | Zhang, H. W., Wang, H., and Wang, J. B. Parametric variational principle based elastic-plastic analysis of materials with polygonal and Voronoi cell finite element methods. Finite Elements in Analysis and Design, 43, 206-217 (2007) |
[27] | Billups, A. C. and Murty, K. C. Complementarity problems. Journal of Computational and Applied Mathematics, 124, 303-318 (2000) |
[28] | Wright, S. J. Primal-Dual Interior-Point Methods, Society for Industrial and Applied Mathematics, Philadelphia (1997) |
[29] | Wen, H., Jin, D. P., and Hu, H. Y. Costate estimation for dynamic systems of the second order. Science in China Series E: Technological Sciences, 52, 752-760 (2009) |
[30] | Iserles, A. and Nørsett, S. P. On the solution of linear differential equations in Lie groups. Philosophical Transactions of the Royal Society A, 357, 983-1019 (1999) |
[31] | Hairer, E., Lubich, C., and Wanner, G. Geometric Numerical Integration: Structure-Preserving Algorithms for Ordinary Differential Equations, Springer, Berlin (2002) |
[32] | Rao, A. V., Benson, D. A., and Darby, C. GPOPS, a MATLAB software for solving multiplephase optimal control problems using the Gauss pseudospectral method. ACM Transactions on Mathematical Software, 37, 1-39 (2010) |
[33] | Bless, R. R. and Hodges, D. H. Finite element solution of optimal control problems with statecontrol inequality constraints. Journal of Guidance, Control, and Dynamics, 15, 1029-1032 (1992) |
[34] | Warner, M. S. and Hodges, D. H. Treatment of control constraints in finite element solution of optimal control problems. Journal of Guidance, Control, and Dynamics, 22, 358-360 (1999) |
[35] | Clohessy, W. H. and Wiltshire, R. S. Terminal guidance for satellite rendezvous. Journal of the Aerospace Sciences, 27, 653-658 (1960) |
[36] | Lawden, D. F. Optimal Trajectories for Space Navigation, Butterworths, London (1963) |
[37] | Kulkarni, J. E., Campbell, M. E., and Dullerud, G. E. Stabilization of spacecraft flight in Halo orbits: an Happroach. IEEE Transactions on Control Systems Technology, 14, 572-578 (2006) |