Article Information
- Yating ZHU, Rubin WANG, Yihong WANG. 2016.
- Impact of θ-burst stimulation on memory mechanism: modeling study
- Appl. Math. Mech. -Engl. Ed., 37(3): 395-402
- http://dx.doi.org/10.1007/s10483-016-2034-6
Article History
- Received Mar. 13, 2015;
- in final form May 29, 2015
Memory, as a higher cognitive function of the brain, has become an important research topic in neural information processing. Three kinds of widely-studied memory are long-term memory, short-term memory, and working memory. Cowan[1] proposed that working memory contained short-term memory, and working memory and short-term memory had a similar neural pro- cessing mechanism. Short-term memory has been maintained to derive from a temporarily activated subset of information in long-term memory, and may decay over time if the acti- vated subset is not refreshed[2, 3]. In the field of neural biology, the long-term potential (LTP) is the best candidate to study the cell mechanism[4], because the LTP has much in common with long-term memory[5]. The stimuli with different properties possess different functions[6, 7]. In a large number of experiments, the θ-burst stimulation (TBS) is used as an LTP induction protocol[8, 9, 10]. The TBS is a repeating pattern of short burst of pulses with brief pauses between the bursts[11]. Previous researches have shown that the magnitude of the LTP can be affected by some factors like frequency, number of pulses, and stimulus intensity. In this work, the TBS is adopted to induce long-term memory on the basis of working memory. Specifically, based on the Camperi-Wang (C-W) model with the Ca2+ subsystem-induced bistability[12], we change the initial stimulus current into the TBS, and assess its impact on the memory mechanism of the model by the control variate method. The researches help to reveal how to form long-term memory with the TBS on the basis of working memory.
2 Model and methodWe adopt the C-W model with the Ca2+ subsystem-induced bistability[12] and the improve- ments of the firing-rate network proposed by Liang et al.[13]. The network consists of N neurons, which are labeled by their preferred cue locations or memory field θi in the range from -π to π. In this limit, the equation of the firing rate r(θi, t) is

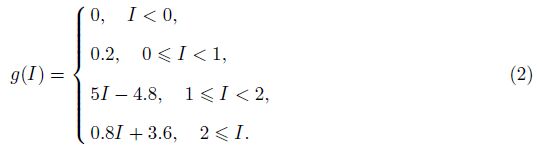
The total input to each neuron I consists of an external input Iext for the transient cue and a recurrent synaptic input Isyn. Since the Ca2+ release might increase the efficacy of the synaptic input, the input I can be represented as follows:

The external input Iext is


The static connectivity weight W between two neurons is given by







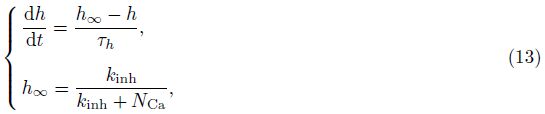


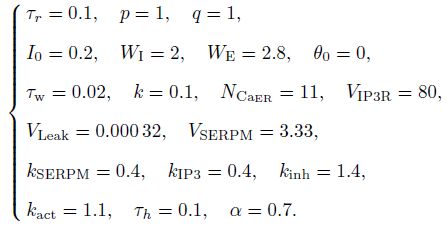
The initial form of the TBS (Icue) studied in this paper is shown in Fig. 1, where t is the time. It consists of ten pulses at 200Hz repeated with 200ms inter-burst-intervals, where the cycle is 5, the amplitude is 1, and the duty ration is 50%. Some parameters remain unchanged, including the stimulus frequency 200Hz, the pulse number of each cycle 10, and the cycle interval 200ms. However, the cycle, amplitude, and duty ration are adjusted according to the control variate method so as to evaluate the impact of these three stimuli properties on the memory model. The starting time is changed to 0 s in the following study.
![]() |
Fig. 1 Initial TBS |
First, set the TBS with the amplitude 1.8 and the duty ration 50%. Comparing the dif- ferences between the network activities under the circumstances of the stimulus cycle 10 (see Fig. 2(a)) and 30 (see Fig. 2 (c)) reveals the impact of the stimulus cycle on the memory per- formance. It is shown that with the increase in the stimulus cycle, the maximum firing rate of the network activities grows and the memory maintaining time delays. From the projections of Figs. 2(a) and 2(c) in the tr-plane (see Figs. 2(b) and 2(d)), we can see that the maximum of the neuron firing rate increases from 6 to 10 and the period of the network activities with the sustained high firing patterns extends 6 s.
![]() |
Fig. 2 Effects of TBS cycle on memory performance |
Then, set the TBS with the cycle 30 and the duty ration 50%. From the differences between the network activities under the circumstances of the stimulus amplitude 1.6 (see Fig. 3(a)) and 1.8 (see Fig. 3(c)), we can see that the effects of the stimulus amplitude on the memory performance can be revealed. From Figs. 3(b) and 3(d), we can see that the maximum of the neuron firing rate increases from 9 to 10 and the period of the network activities with the sustained high firing patterns extends from 21 s to 22 s.
![]() |
Fig. 3 Effects of TBS amplitude on memory performance |
At last, set the TBS with the cycle 30 and the amplitude 1.4. From the differences between the network activities under the circumstances of the stimulus duty ration 65% (see Fig. 4(a)) and 80% (see Fig. 4(c)), we can see that the effects of the stimulus amplitude on the memory performance can be revealed. From Fig. 4(d), we can see that the peak of the neuron firing rate exceeds 12 and sustains a high firing rate for 2 s after reaching the peak and the memory time exceeds 4 s (see Figs. 4(b) and 4(d)).
![]() |
Fig. 4 Effects of TBS duty ration on memory performance |
The study shows the adjustment of the comprehensive effect of three kinds of stimuli prop- erties, i.e., cycle, amplitude, and duty ration, of the TBS and that working memory can be transformed to long-term memory. First, set the stimulus properties as the cycle 30, the am- plitude 1.8, and the duty ration 50%, and get the network firing pattern (see Fig. 5(a)) and its corresponding projection in the tr-plane (see Fig. 5(b)). It can be seen that the memory time is 21 s, belonging to working memory. Then, set the stimulus properties as the cycle 30, the amplitude 1.9, and the duty ration 50%, and get Figs. 5(c) and 5(d). It is shown that the memory time is 70 s, meeting the requirement of long-term memory. That is to say, when the stimulus is set as the cycle 30 and the duty ration 50%, the amplitude 1.9 is the critical value to produce long-term memory.
![]() |
Fig. 5 Effects of TBS cycle on memory performance |
The specific stimuli is used in a working memory model to produce long-term memory. The improved C-W model with the Ca2+ subsystem-induced bistability is adopted, and the changes of the memory mode in this model are studied under the adjustment of the properties such as cycle, amplitude, and duty ration of the TBS. The research shows that changing the network firing pattern has an effect to some extent on the memory maintaining time. Increasing the cycle, amplitude, and duty ration will increase the firing rate of the network activities and make the memory last longer. When the TBS is set as cycle 30 and duty ration 50%, and the amplitude increases to 1.9, the working memory model can be activated to produce long-term memory. The mechanism of memory formation can be further studied on the basis of our theoretical analysis.
[1] | Cowan, N. What are the differences between long-term, short-term, and working memory? Progress in Brain Research, 169, 323-338 (2008) |
[2] | Cowan, N. Evolving conceptions of memory storage, selective attention, and their mutual con- straints within the human information processing system. Psychology Bulletin, 104, 163-191 (1988) |
[3] | Cowan, N. The magical number 4 in short-term memory: a reconsideration of mental storage capacity. Behavior in Brain Science, 24, 87-185 (2001) |
[4] | Timothee, L. and Kazuyuki, A. Combined effects of LTP/LTD and synaptic scaling in forma- tion of discrete and line attractors with persistent activity from non-trivial baseline. Cognitive Neurodynamics, 6, 499-524 (2012) |
[5] | Cooke, S. F. and Bliss, T. V. Plasticity in the human central nervous system. Brain, 129, 1659- 1673 (2006) |
[6] | Seiya, I. and Yoshio, S. Recall of sequences based on the position of the first cue stimulus in rats. Cognitive Neurodynamics, 8, 345-351 (2014) |
[7] | Lars, H., Carlos, T., Mai, M., and Daniel, J. S. Neurofunctional model of large-scale correlates of selective attention governed by stimulus-novelty. Cognitive Neurodynamics, 5, 104-111 (2011) |
[8] | Jennifer, A. S., Katharine, C. E., Charles, N. M., and Jonathan, P. M. Improved learning and memory with theta-burst stimulation of the fornix in rat model of traumatic brain injury. Hippocampus , 24, 1-8 (2014) |
[9] | Otto, T., Eichenbaum, H., Wiener, S. I., and Wible, C. G. Learning-related patterns of CA1 spike trains parallel stimulation parameters optimal for inducing hippocampal long-term potentiation. Hippocampus, 1, 181-192 (1991) |
[10] | Yun, S. H., Mook-Jung, I., and Jung, M.W. Variation in effective stimulus patterns for induction of long-term potentiation across different layers of rat entorhinal cortex. The Journal of Neuroscience, 22, 1-5 (2002) |
[11] | Perez, Y., Chapman, C. A., Woodhall, G., Robitaille, R., and Lacaille, J. C. Differential induction of long-lasting potentiation of inhibitory postsynaptic potentials by theta patterned stimulation versus 100-Hz tetanization in hippocampal pyramidal cells in vitro. Neuroscience, 90, 747-757 (1999) |
[12] | Fall, C. P. and Rinzel, J. An intracellular Ca2+ subsystem as a biologically plausible source of intrinsic conditional bi-stability in a network model of working memory. Journal of Computational Neuroscience, 20, 97-107 (2006) |
[13] | Liang, L., Wang, R., and Zhang, Z. The modeling and simulation of visuospatial working memory. Cognitive Neurodynamics, 4, 359-366 (2010) |
[14] | Wang, R. B., Shen, E. H., and Gu, F. J. Advances in cognitive neurodynamics ICCN 2007. Proceedings of the International Conference on Cognitive Neurodynamics, Springer, Heidelberg, 463-467 (2008) |
[15] | Shriki, O., Hansel, D., and Sompolinsky, H. Rate models for conductance-based cortical beural networks. Neural Computation, 15, 1809-1841 (2003) |
[16] | Fall, C. P. and Rinzel, J. An intracellular Ca2+ subsystem as a biologically plausible source of in- trinsic conditional bi-stability in a network model of working memory. Computatinal Neuroscience, 20, 97-107 (2006) |
[17] | Eugen, T. Short term memory may be the depletion of the readily releasable pool of presynaptic neurotransmitter vesicles of a metastable long term memory trace pattern. Cognitive Neurodynamics , 3, 263-269 (2009) |